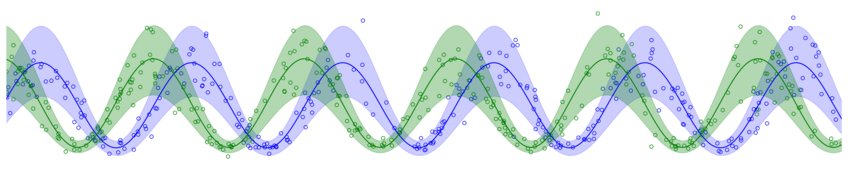
QS_II | TORCH: Towards operational uncertainty characterisation for high-resolution AI based biogenic CO2 fluxes
TORCH complements tasks in WP-Q&S_I.3 ("Biogenic Fluxes") regarding biogenic CO2 fluxes based on machine learning with respect to a thorough assessment of the uncertainties of the generated products.
The expected outcome is the introduction of automated tools that perform a series of complementary uncertainty assessments so that objective and quantitative information on error structures of the biogenic prior can become available in the future operational context of ITMS. This will facilitate an optimal integration of the AI-based biogenic priors with atmospheric inversions in ITMS-M.
Our approach to assessing uncertainties and errors of biogenic CO2 flux products for ITMS combines three complementary perspectives to address different aspects of uncertainty:
- a consistent leave-station-out cross-validation, where the predictions are analysed for their errors when station data have been excluded from training;
- the creation of an ensemble of products resulting from different methodological sources of uncertainty;
- consistency checks with independently derived CO2 flux estimates, amongst others for specific sectors (agriculture and forestry) in Germany based on national inventories and specific process model simulations for Germany, which will become available in the Q&S module
Participating institutions:
- Max Planck Institute for Biogeochemistry, Global Diagnostic Modelling Group
Involved persons:
